Model Evaluation¶
import random
import tensorflow as tf
import matplotlib.pyplot as plt
import sklearn
import numpy as np
from PIL import Image
from tensorflow.examples.tutorials.mnist import input_data
/home/ma-user/anaconda3/envs/TensorFlow-1.13.1/lib/python3.6/site-packages/tensorflow/python/framework/dtypes.py:526: FutureWarning: Passing (type, 1) or '1type' as a synonym of type is deprecated; in a future version of numpy, it will be understood as (type, (1,)) / '(1,)type'.
_np_qint8 = np.dtype([("qint8", np.int8, 1)])
/home/ma-user/anaconda3/envs/TensorFlow-1.13.1/lib/python3.6/site-packages/tensorflow/python/framework/dtypes.py:527: FutureWarning: Passing (type, 1) or '1type' as a synonym of type is deprecated; in a future version of numpy, it will be understood as (type, (1,)) / '(1,)type'.
_np_quint8 = np.dtype([("quint8", np.uint8, 1)])
/home/ma-user/anaconda3/envs/TensorFlow-1.13.1/lib/python3.6/site-packages/tensorflow/python/framework/dtypes.py:528: FutureWarning: Passing (type, 1) or '1type' as a synonym of type is deprecated; in a future version of numpy, it will be understood as (type, (1,)) / '(1,)type'.
_np_qint16 = np.dtype([("qint16", np.int16, 1)])
/home/ma-user/anaconda3/envs/TensorFlow-1.13.1/lib/python3.6/site-packages/tensorflow/python/framework/dtypes.py:529: FutureWarning: Passing (type, 1) or '1type' as a synonym of type is deprecated; in a future version of numpy, it will be understood as (type, (1,)) / '(1,)type'.
_np_quint16 = np.dtype([("quint16", np.uint16, 1)])
/home/ma-user/anaconda3/envs/TensorFlow-1.13.1/lib/python3.6/site-packages/tensorflow/python/framework/dtypes.py:530: FutureWarning: Passing (type, 1) or '1type' as a synonym of type is deprecated; in a future version of numpy, it will be understood as (type, (1,)) / '(1,)type'.
_np_qint32 = np.dtype([("qint32", np.int32, 1)])
/home/ma-user/anaconda3/envs/TensorFlow-1.13.1/lib/python3.6/site-packages/tensorflow/python/framework/dtypes.py:535: FutureWarning: Passing (type, 1) or '1type' as a synonym of type is deprecated; in a future version of numpy, it will be understood as (type, (1,)) / '(1,)type'.
np_resource = np.dtype([("resource", np.ubyte, 1)])
sess = tf.Session()
mnist = input_data.read_data_sets("MINST_data", one_hot=True)
WARNING:tensorflow:From <ipython-input-2-7da412c0683a>:2: read_data_sets (from tensorflow.contrib.learn.python.learn.datasets.mnist) is deprecated and will be removed in a future version.
Instructions for updating:
Please use alternatives such as official/mnist/dataset.py from tensorflow/models.
WARNING:tensorflow:From /home/ma-user/anaconda3/envs/TensorFlow-1.13.1/lib/python3.6/site-packages/tensorflow/contrib/learn/python/learn/datasets/mnist.py:260: maybe_download (from tensorflow.contrib.learn.python.learn.datasets.base) is deprecated and will be removed in a future version.
Instructions for updating:
Please write your own downloading logic.
WARNING:tensorflow:From /home/ma-user/anaconda3/envs/TensorFlow-1.13.1/lib/python3.6/site-packages/tensorflow/contrib/learn/python/learn/datasets/mnist.py:262: extract_images (from tensorflow.contrib.learn.python.learn.datasets.mnist) is deprecated and will be removed in a future version.
Instructions for updating:
Please use tf.data to implement this functionality.
Extracting MINST_data/train-images-idx3-ubyte.gz
WARNING:tensorflow:From /home/ma-user/anaconda3/envs/TensorFlow-1.13.1/lib/python3.6/site-packages/tensorflow/contrib/learn/python/learn/datasets/mnist.py:267: extract_labels (from tensorflow.contrib.learn.python.learn.datasets.mnist) is deprecated and will be removed in a future version.
Instructions for updating:
Please use tf.data to implement this functionality.
Extracting MINST_data/train-labels-idx1-ubyte.gz
WARNING:tensorflow:From /home/ma-user/anaconda3/envs/TensorFlow-1.13.1/lib/python3.6/site-packages/tensorflow/contrib/learn/python/learn/datasets/mnist.py:110: dense_to_one_hot (from tensorflow.contrib.learn.python.learn.datasets.mnist) is deprecated and will be removed in a future version.
Instructions for updating:
Please use tf.one_hot on tensors.
Extracting MINST_data/t10k-images-idx3-ubyte.gz
Extracting MINST_data/t10k-labels-idx1-ubyte.gz
WARNING:tensorflow:From /home/ma-user/anaconda3/envs/TensorFlow-1.13.1/lib/python3.6/site-packages/tensorflow/contrib/learn/python/learn/datasets/mnist.py:290: DataSet.__init__ (from tensorflow.contrib.learn.python.learn.datasets.mnist) is deprecated and will be removed in a future version.
Instructions for updating:
Please use alternatives such as official/mnist/dataset.py from tensorflow/models.
train_size = len(mnist.train.images)
test_size = len(mnist.test.images)
rand_train_indices = np.random.choice(len(mnist.train.images), train_size, replace=False)
rand_test_indices = np.random.choice(len(mnist.test.images), test_size, replace=False)
x_vals_train = mnist.train.images[rand_train_indices]
x_vals_test = mnist.test.images[rand_test_indices]
y_vals_train = mnist.train.labels[rand_train_indices]
y_vals_test = mnist.test.labels[rand_test_indices]
k = 10
batch_size = 6
x_data_train = tf.placeholder(shape=[None,784], dtype=tf.float32)
x_data_test = tf.placeholder(shape=[None,784], dtype=tf.float32)
y_target_train = tf.placeholder(shape=[None,10],dtype=tf.float32)
y_target_test = tf.placeholder(shape=[None, 10], dtype=tf.float32)
distance = tf.reduce_sum(tf.abs(tf.subtract(x_data_train, tf.expand_dims(x_data_test, 1))),reduction_indices=2)
top_k_xvals, top_k_indices = tf.nn.top_k(tf.negative(distance),k=k)
prediction_indices = tf.gather(y_target_train, top_k_indices)
count_of_predictions = tf.reduce_sum(prediction_indices, reduction_indices=1)
prediction = tf.argmax(count_of_predictions, dimension=1)
WARNING:tensorflow:From <ipython-input-5-638de782a748>:4: calling argmax (from tensorflow.python.ops.math_ops) with dimension is deprecated and will be removed in a future version. Instructions for updating: Use the axis argument instead
num_loops = int(np.ceil(len(x_vals_test)/batch_size))
test_output = []
actual_vals = []
for i in range(num_loops):
min_index = i*batch_size
max_index = min((i+1)*batch_size, len(x_vals_train))
x_batch = x_vals_test[min_index:max_index]
y_batch = y_vals_test[min_index: max_index]
predictions = sess.run(prediction,feed_dict={x_data_train: x_vals_train, x_data_test: x_batch, y_target_train: y_vals_train, y_target_test: y_batch})
test_output.extend(predictions)
actual_vals.extend(np.argmax(y_batch, axis=1))
accuracy = sum([1./test_size for i in range(test_size) if test_output[i]==actual_vals[i]])
print("Accuracy on test set: " + str(accuracy))
Accuracy on test set: 0.9586999999999107
actuals = np.argmax(y_batch, axis=1)
Nrows = 1
Ncols = 4
for i in range(len(actuals)):
plt.subplot(Nrows, Ncols, i+1)
plt.imshow(np.reshape(x_batch[i], [28,28]), cmap="Greys_r")
plt.title("Acutal: " + str(actuals[i]) + " " + "Pred: " + str(predictions[i]), fontsize=10)
frame = plt.gca()
frame.axes.get_xaxis().set_visible(False)
frame.axes.get_yaxis().set_visible(False)
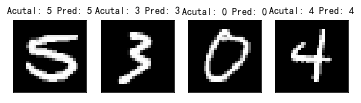